Bias Vs Variance
Bias Vs Variance Tradeoff
Bias is a metric used to evaluate a machine learning model’s ability to learn from the training data. A model with high bias will therefore not perform well on both the training data nor on test data presented to it. Models with low bias, conversely, learn well from the presented training data.
Variance is a metric used to evaluate the ability of the trained model to generalize to some test dataset. More broadly, it also represents how similar the results from a model will be if it were fed different data from the same process.
Models with low bias (which can learn from the training data well) often have high variance (and therefore an inability to generalize to new data), and this phenomenon is referred to as “overfitting”. By definition, therefore, high model variance despite low model bias is referred to as overfitting.
How to Prevent Overfitting
Cross-validation
Cross-validation is a powerful preventative measure against overfitting.The idea is clever: Use your initial training data to generate multiple mini train-test splits. Use these splits to tune your model. In standard k-fold cross-validation, we partition the data into k subsets, called folds. Then, we iteratively train the algorithm on k-1 folds while using the remaining fold as the test set (called the “holdout fold”).
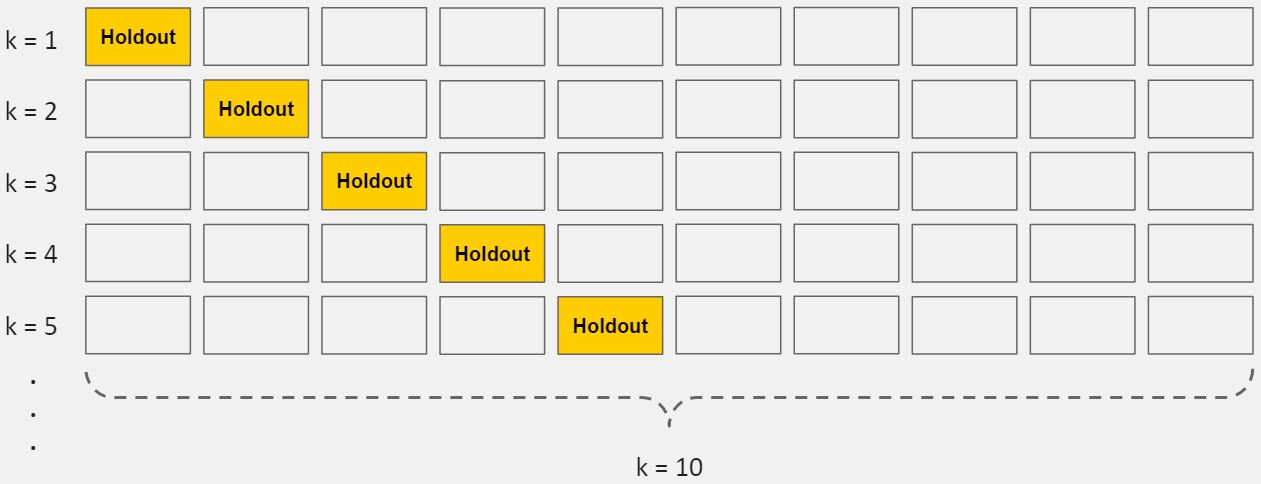
Remove features
Some algorithms have built-in feature selection. For those that don’t, you can manually improve their generalizability by removing irrelevant input features.
Train with more data
It won’t work every time, but training with more data can help algorithms detect the signal better. In the earlier example of modeling height vs. age in children, it’s clear how sampling more schools will help your model.
Early stopping
When you’re training a learning algorithm iteratively, you can measure how well each iteration of the model performs. Up until a certain number of iterations, new iterations improve the model. After that point, however, the model’s ability to generalize can weaken as it begins to overfit the training data. Early stopping refers stopping the training process before the learner passes that point.
Regularization
Regularization refers to a broad range of techniques for artificially forcing your model to be simpler. The method will depend on the type of learner you’re using. For example, you could prune a decision tree, use dropout on a neural network, or add a penalty parameter to the cost function in regression. Oftentimes, the regularization method is a hyperparameter as well, which means it can be tuned through cross-validation.
Ensembling
Ensembles are machine learning methods for combining predictions from multiple separate models. There are a few different methods for ensembling, but the two most common are:
Bagging attempts to reduce the chance overfitting complex models.
- It trains a large number of "strong" learners in parallel.
- A strong learner is a model that's relatively unconstrained.
- Bagging then combines all the strong learners together in order to "smooth out" their predictions.
Boosting attempts to improve the predictive flexibility of simple models.
- It trains a large number of "weak" learners in sequence.
- A weak learner is a constrained model (i.e. you could limit the max depth of each decision tree).
- Each one in the sequence focuses on learning from the mistakes of the one before it.
- Boosting then combines all the weak learners into a single strong learner.
Comments
Post a Comment